Discover key insights from the recent online scheduling seminar, where Ender Ozcan from the University of Nottingham discussed the integration of Machine Learning with Hyper-heuristics. This event, supported by ROBOPROX and organized by Zdeněk Hanzálek, Michael Pinedo, and Guohua Wan, highlighted cutting-edge advancements in optimization techniques.
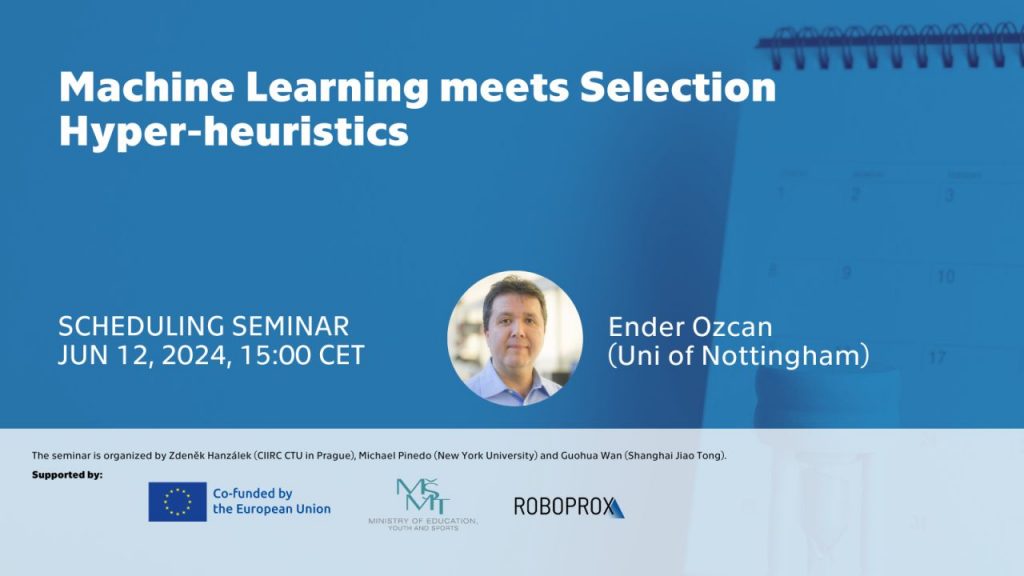
Hyper-heuristics, as elucidated by Ozcan, are potent search methodologies that leverage low-level heuristics or heuristic components to address computationally demanding optimization problems. This approach encompasses two primary types: selection and generation hyper-heuristics. Selection hyper-heuristics involve intelligently choosing and applying a suitable heuristic at each step of the search process, while generation hyper-heuristics automate the construction of heuristics or heuristic components during optimization.
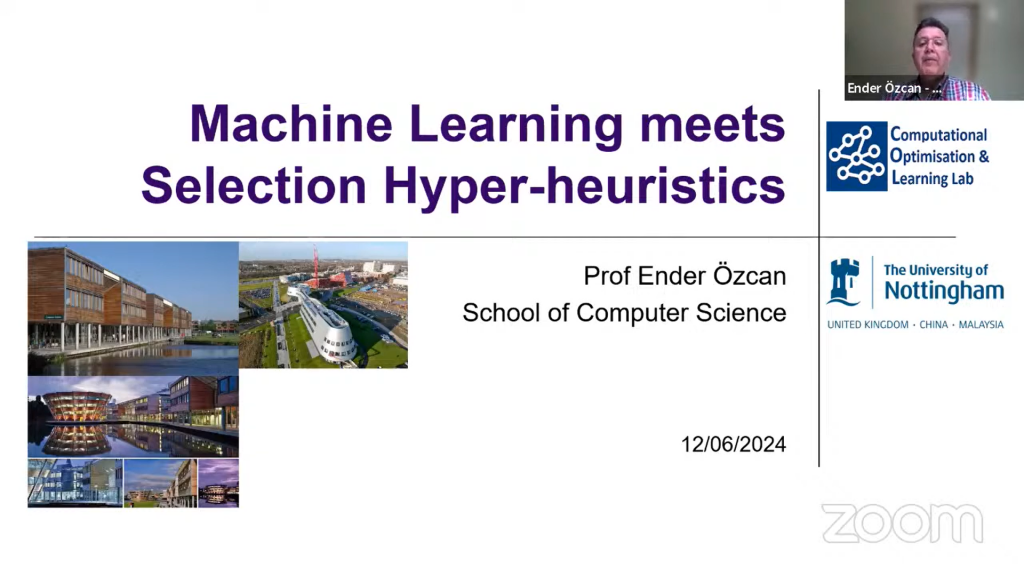
Ozcan’s presentation provided a concise overview of these methodologies, emphasizing their roles in enhancing computational efficiency and solution quality across various domains. He showcased illustrative case studies that demonstrate the application of Machine Learning techniques to design more effective selection hyper-heuristics autonomously.
For those interested in delving deeper into the seminar’s topic, information are available on the website. You can also find the recording of the entire seminar here.