Within the ROBOPROX Project, we would like to invite you to the seminar:
Design Optimization via the Continuous Stochastic Gradient Method
by Prof. Dr. Michael Stingl, Chair of Applied Mathematics (Continuous Optimization), Department of Mathematics, Friedrich-Alexander-Universität Erlangen-Nürnberg who will present joint research work in collaboration with Lukas Pflug and Andrian Uihlein-
1 October 2024, 10:00-11:00 CET
Faculty of Civil Engineering CTU in Prague
Thákurova 7, Prague 6 | Room B-168
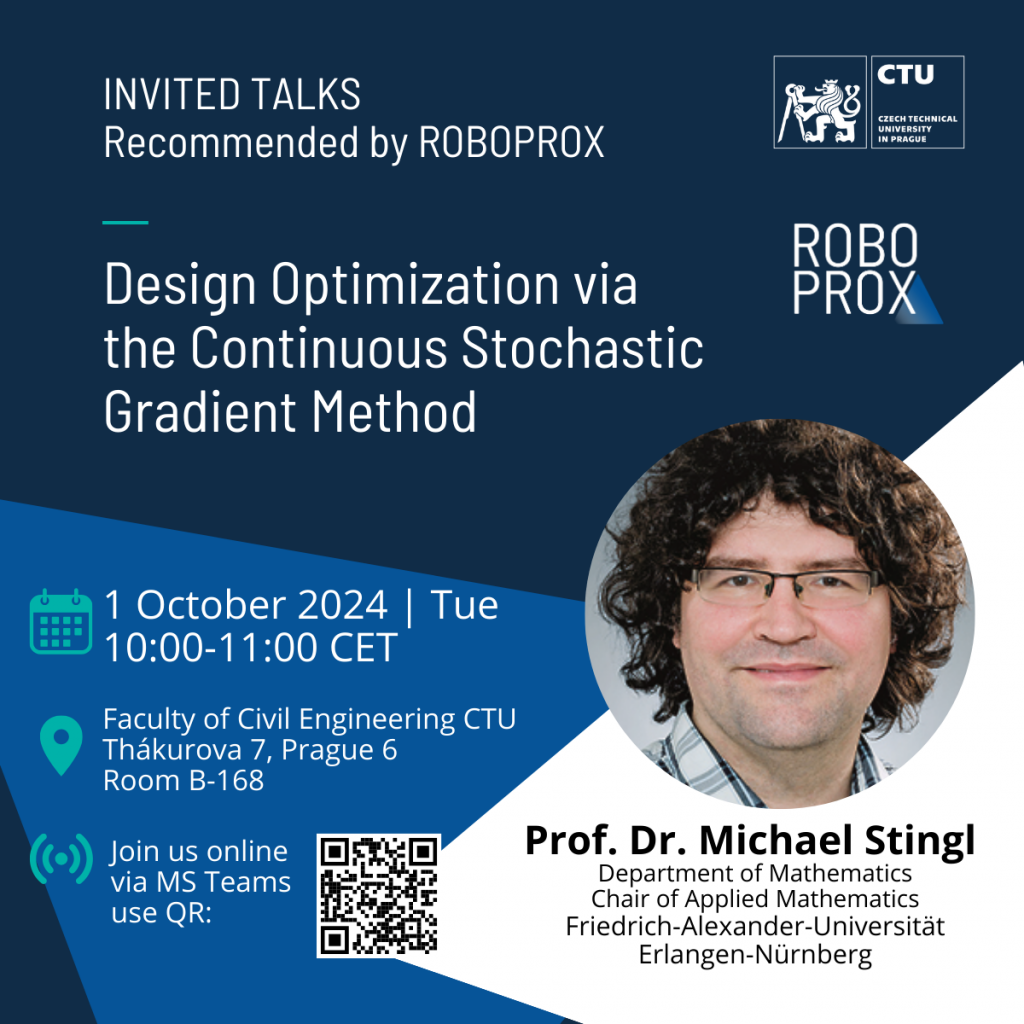
Abstract:
We consider large-scale optimization problems with objective functions or constraints depending on random or distributed parameters. Important examples are problems in which expected properties are minimized or problems subject to chance constraints. Here, standard deterministic optimization approaches rely on a discretization of the appearing integrals. However, the underlying quadrature rule can introduce artificial local minima, resulting in an overall poor performance of the optimizer. Since circumventing this effect severely increases the computational cost, stochastic gradient-type methods have gained popularity over the years, most recently also for the solution of topology optimization problems. However, standard schemes from the literature are typically limited to expected loss functions and still require many iterations. This implies that a lot of expensive state problems have to be solved.
Thus, we present the continuous stochastic gradient method (CSG), which provides an efficient hybrid approach without these limitations. In CSG, samples calculated in previous iterations are collected in an optimal linear combination to obtain an approximation to the full gradient and objective function value. It can be shown that the approximation error for both quantities vanishes during the optimization process. Therefore, CSG inherits many convergence properties known from full gradient methods, like convergence for constant step sizes. Moreover, the approximation idea in CSG is not limited to simple gradient schemes but can be combined with more elaborated sequential programming techniques. As an example, the combination with the well-known MMA scheme is shown. After presenting the main theoretical properties of CSG, its efficiency is demonstrated for applications from elasticity and/or nano-particle optics.
This presentation was supported by the Ministry of Education, Youth, and Sports of Czechia through projects 8J24DE005 (Free-material optimization for manufacturable modular structures) and CZ.02.01.01/00/22_008/0004590 (ROBOPROX).